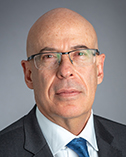
Juan J. de Pablo
The University of Chicago
Primary Section: 14, Chemistry Secondary Section: 31, Engineering Sciences Membership Type:
Member
(elected 2022)
|
Biosketch
Juan de Pablo is a chemical engineer known for his work in theoretical and computational modeling of macromolecular systems and complex fluids. His work cuts across length and time scales, starting from the atomic level to the macroscopic. Some of the models, theories, and computational methods that he has developed are widely used for the study and design of molecules and materials systems. De Pablo grew up in Mexico City, and he graduated from the National Autonomous University of Mexico with a BS degree in engineering. He then received a PhD in chemical engineering from the University of California – Berkeley. He was a postdoctoral fellow at the Polymer Institute of the ETH in Zurich, Switzerland, prior to joining the University of Wisconsin – Madison as an Assistant Professor. In 2012, de Pablo became the Liew Family Professor of Molecular Engineering at the University of Chicago, where he now serves as the Executive Vice President for Science Strategy, Innovation, National Laboratories and Global Initiatives. De Pablo is a member of the American Academy of Arts and Sciences, the National Academy of Engineering, the National Academy of Sciences, and the Mexican Academy of Sciences.
Research Interests
Juan de Pablo’s research and scholarly interests are focused on macromolecular systems, including polymers, DNA and RNA, proteins, and liquid crystals. His work entails developing molecular and multi-scale models of materials, which are used to understand their function and to design new systems for specific applications. He has developed a broad range of theoretical and computational frameworks, as well as mathematical algorithms, that are widely used for the study of condensed matter. A cross-cutting and critical aspect of this work has been to establish well-defined connections between molecular characteristics and macroscopic behavior. In recent years, a growing emphasis has been to conceive new strategies for molecular and materials discovery that rely on emerging concepts from machine-learning and artificial intelligence, extensive experimental and computational data sets, and a combination of physics, chemistry, and engineering-based models. These strategies are have led to advances in a range of fields, such as drug development, epigenetics, defect control in advanced materials, and active matter, to name a few.